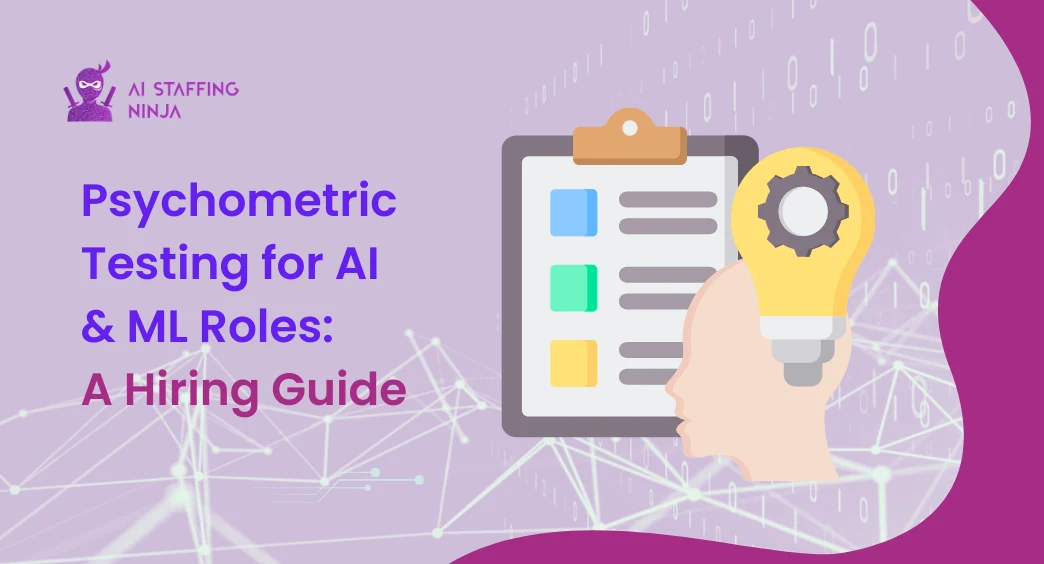
- By : By Staffing Ninja
Why Pre-Employment Assessments Matter for Quality AI/ML Hires
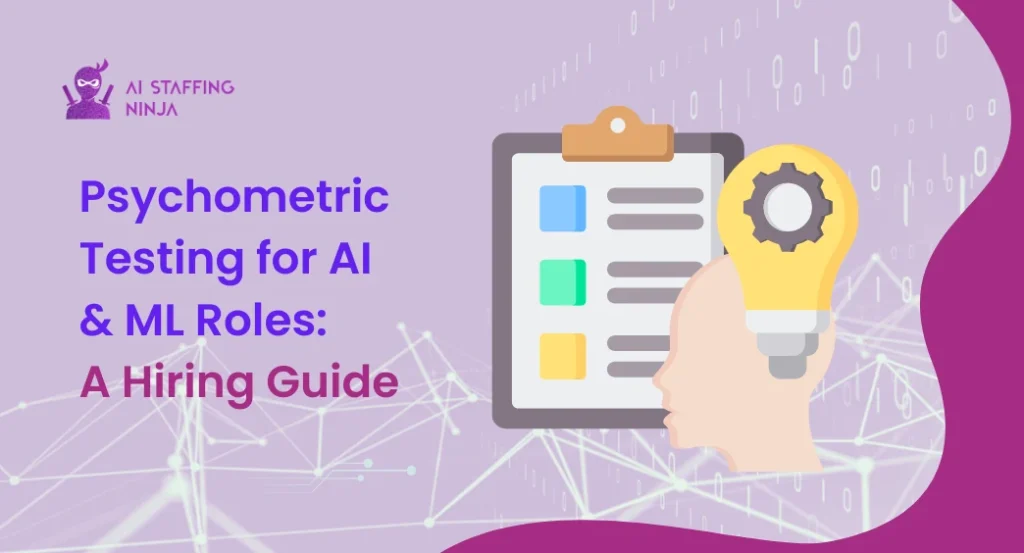
Artificial intelligence and machine learning are experiencing significant growth; however, finding top AI/ML talent presents a different challenge. Studies show that 89% of hiring failures result from poor job fit and skill gaps rather than a weak resume. Traditional hiring methods, such as scanning CVs and conducting generic interviews, often fail to assess genuine expertise accurately.
The solution? Pre-employment assessments. These structured evaluations help every AI recruitment agency identify the best candidates based on real skills, not just impressive resumes. By using data-driven hiring techniques, companies can build stronger AI/ML teams while reducing hiring risks.
What Are Pre-Employment Assessments?
Pre-employment assessments are tests intended to evaluate a candidate’s cognitive abilities, technical skills, and behavioral traits prior to employment. In AI/ML hiring, these tests ensure that candidates have the expertise needed to thrive in complex roles.
Here are the key types of assessments:
- Technical Assessments – These tests evaluate a candidate’s coding skills, machine learning model development, and proficiency in programming languages. They help AI staffing teams verify hands-on expertise.
- Cognitive Ability Tests – AI professionals need strong problem-solving skills. These tests measure logical reasoning and critical thinking—essential for working with complex algorithms.
- Behavioral Assessments – AI/ML teams require more than just technical expertise. These tests assess soft skills such as teamwork and communication, ensuring candidates can work effectively in collaborative environments.
By employing these assessments, AI recruitment solutions can make data-driven recruiting decisions and mitigate the likelihood of mis-hires.
How Pre-Employment Assessments Improve AI/ML Hiring
Skills-based hiring is far more reliable than evaluating resumes alone. Structured pre-employment tests offer several advantages:
- Identify Real Expertise – Many candidates can list AI/ML skills on their resumes, but organized testing demonstrates their ability to apply them. These tests identify top performers with practical skills.
- Reduce Hiring Bias – AI recruitment agencies often struggle with unconscious bias during hiring. Objective assessments ensure that candidates are evaluated based on skills rather than education or personal connections.
- Improve Diversity in AI/ML Teams – When companies focus on skills rather than credentials, they gain access to a larger talent pool. This leads to more diverse and innovative AI teams.
With AI hiring becoming increasingly competitive, pre-employment assessments help organizations identify the best-fit candidates quickly and efficiently.
Key Assessments for AI/ML Candidates
To hire top-tier AI/ML professionals, AI recruitment companies should use a mix of technical and soft-skill assessments.
- Coding Challenges: These tests evaluate a candidate’s proficiency in programming languages like Python, R, or Java and frameworks such as TensorFlow and PyTorch.
- Algorithm & Data Structure Tests: Strong AI engineers need deep problem-solving abilities. These tests measure how well candidates understand and implement algorithms, which is crucial for AI/ML development.
- Machine Learning Case Studies: Real-world problem-solving is essential in AI/ML roles. Candidates may be asked to analyze datasets, build predictive models, or optimize an AI solution based on a specific business challenge.
- Behavioral & Soft Skills Tests: AI professionals often work in teams and interact with different departments. Testing for communication, collaboration, and adaptability ensures a candidate is a good cultural fit.
How AI Companies Benefit from Pre-Employment Assessments
AI recruitment is expensive, and a bad hire may cost companies thousands of dollars in lost productivity. Pre-employment assessments help AI staffing firms in the following ways:
- Reducing Mis-Hires: Hiring mistakes are costly, especially in specialized fields like AI/ML. Structured assessments help filter out underqualified candidates before they join the team.
- Improving Hiring Efficiency: Instead of spending hours screening resumes and conducting multiple interviews, companies can fast-track top candidates based on their test results.
- Lowering Hiring Costs: AI recruitment agencies and in-house teams save time and resources by identifying top candidates early in the process, reducing the need for lengthy interview cycles.
Common Mistakes to Avoid with Pre-Employment Testing
Pre-employment assessments are powerful tools, but to get the best results, you must use them correctly. Here are some common mistakes companies make:
- Relying on Only One Type of Test: Just testing coding skills is insufficient. AI/ML roles require problem-solving, creativity, and teamwork, so a mix of technical and behavioral tests is essential.
- Setting Unrealistic Difficulty Levels: While it’s important to challenge candidates, overly complex tests can discourage even strong applicants. Tests should reflect real job requirements, not just theoretical knowledge.
- Ignoring Soft Skills: Many companies focus solely on technical assessments and overlook crucial soft skills. An AI engineer who is unable to collaborate effectively can slow down the entire team.
Strengthening AI/ML Hiring with Pre-Employment Assessments
AI/ML hiring is more competitive than ever, and traditional methods are no longer enough. Pre-employment assessments provide a structured, data-driven approach to hiring, ensuring companies find the best talent without guesswork.
Using technical, cognitive, and behavioral assessments, organizations can reduce mishires, improve team efficiency, and lower hiring costs. If your company is looking to hire AI/ML professionals, it’s time to integrate structured assessments into your recruitment process for better, smarter AI and machine learning hiring decisions.