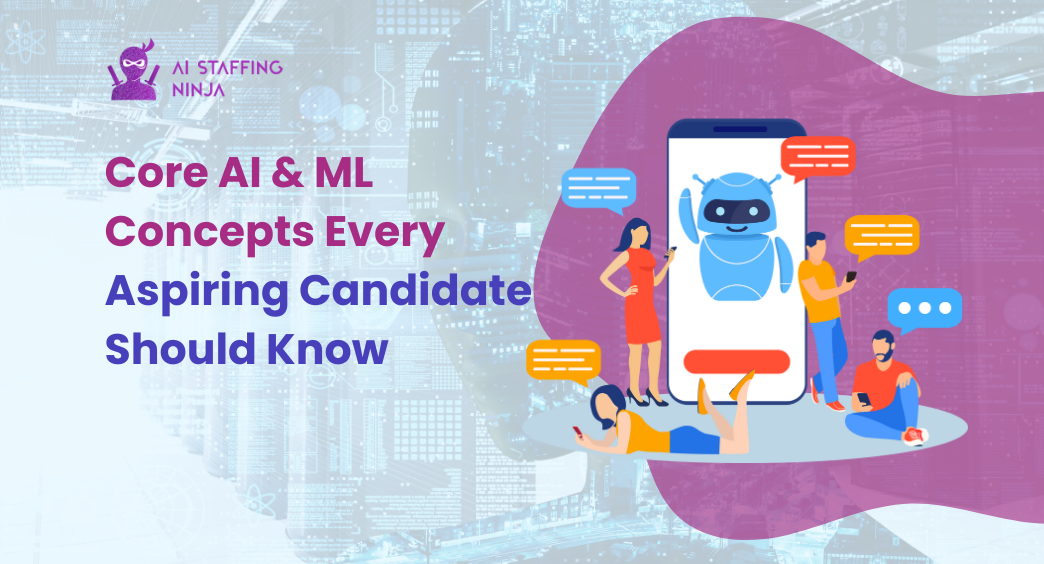
- By : By AI Staffing Ninja
Understanding the Basics of Artificial Intelligence and Machine Learning
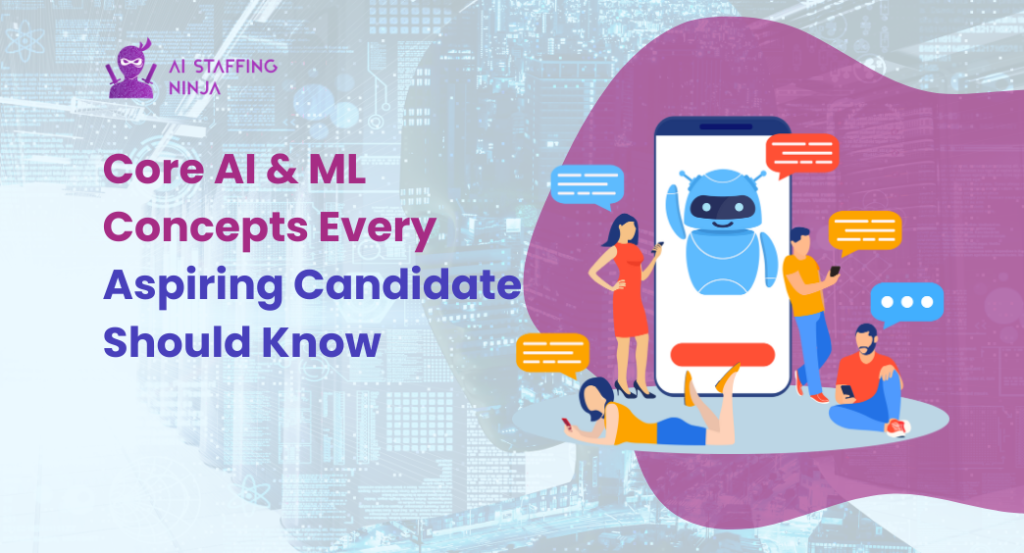
The scope of this article is to elucidate the basics of artificial intelligence (AI) and machine learning (ML) for readers who are aliens with these revolutionary topics. AI and ML have risen to be an integral part of widespread domains in day-to-day life, such that their presence brings forth a well-pronounced dynamic transformation. Therefore, a basic understanding of AI and ML expounds their potential in metamorphosing existing applications into a refined one endowed with deceiving attributes. Let’s delve into its fundamentals for better insights
Defining Artificial Intelligence
- What is Artificial Intelligence?: AI refers to the potential of computational systems to simulate the widespread tasks oriented with human intelligence, including human learning, decision-making, problem-solving, autonomy, and creativity. The history of AI rolls back to 1950 when Alan Turing coined the term artificial intelligence and presented it as a theoretical concept in his paper, “Computing Machinery and Intelligence.” A couple of graduate students picked this idea, related it to Warren and Walter’s (1943) suggested model of artificial neurons, and evolved with the first neural net machine, SNARC, in 1951. Today, AI creates waves in every domain, including healthcare and financial services.
Understanding Machine Learning
- What is Machine Learning?: ML is a subset of AI that concentrates on computer algorithms that facilitate machines to learn and upgrade from data analysis over time without distinct programming methodologies. It deals with making machines imitate human learning, behave autonomously, and intensify their performance and precision through experience and exposure to extensive data.
- Relationship Between AI and ML: AI features the study, design, and development of systems that accomplish human-accomplishable tasks. It achieves this by being trained on datasets that comprise the instructions to achieve them, on which the ML works. ML is a part of AI, and not all AI involves ML.
Types of Artificial Intelligence
- Narrow AI (Weak AI): This type of AI finds significance in narrow tasks like internet searches, facial recognition, or driving a car. It operates in a restricted, pre-determined set of contexts. Widespread AI systems, including those that play complicated games like chess and Go, fall under this type.
- General AI (Strong AI): This category is bestowed with extensive humanist cognitive traits, empowered to subconsciously manage new and strange tasks. However, this idea is still under research.
- Superintelligent AI: This refers to a futuristic AI version in which machines could possibly transcend human intelligence over widespread attributes like creativity, wisdom, and problem-solving. This is still speculative and awaiting recognition.
Types of Machine Learning
- Supervised Learning: In this type of ML, the model is trained from a labeled dataset consisting of input-output pairs. Every dataset input is labeled to its corresponding output, which facilitates making predictions on fresh, unseen data. Its categories include regression and classification.
- Unsupervised Learning: This ML type utilizes the input data with unlabeled responses to reveal the data’s hidden structures. These learning algorithms attempt to directly learn patterns and relationships from the input data. Clustering is one common unsupervised learning technique.
- Reinforcement Learning: Here, the agents accomplish decision-making by completing actions and measuring outcomes through penalties or rewards. The chess game is a classical example of reinforcement learning. The RL can either be model-based, model-free, or partially observable.
Key Concepts and Terminology
- Algorithms: Algorithms are the set of instructions the AI system ought to follow. They form the “brain” of AI systems as they escort the calculations, data processing, and decision-making processes, and hence, they are necessary for both AI and ML.
- Neural Networks: Neural networks are computational models inspired by the human brain. They are used in both AI and ML for tasks like recognizing patterns, classifying data, and decision-making (e.g., for image or speech recognition).
- Deep Learning: Deep learning is a subset of machine learning that uses deep neural networks (many layers of neural networks). With numerous hidden layers, deep learning algorithms analyze complex patterns in large datasets, like images, video, and text.
- Overfitting/Underfitting: The AI model is termed overfitting when it performs overwhelmingly well for training data but underperforms for new test data. It is also called overfitting when it hasn’t comprehended the patterns in training data and hence fails to handle new data.
- Data Mining: Data mining is the process of extracting useful patterns, knowledge, or information from a varied range of datasets. ML utilizes these patterns to train AI models to handle tasks without requiring elaborate instructions. AI systems employ data mining to comprehend, interpret, and work with the data to make data-driven predictions, decisions, and insights.
Applications of AI and ML
Healthcare: The basics of machine learning and AI are rudimentary in diagnosing diseases, scheming personalized treatment plans, and maintaining data like patient records, medical images, and genetic details.
Finance: Finance domain employs AI and ML in detecting fraudulent activities, enhancing security, improving customer service, optimizing operations, and in algorithmic trading.
Transportation: By understanding machine learning, the automotive industry employs ML and AI to develop self-driven vehicles with precise computer vision and traffic management systems.
Everyday Life: The role of chatbots and virtual assistants like Siri and Alexa powered by NLP are witnesses of AI and ML impact in everyday life. The recommendation systems employed in streaming services are yet another worth mention.
The Future of AI and ML
Emerging Trends: By insightfully understanding artificial intelligence has evolved into mind-blowing developments in robots’ potential to learn by cautiously observing human actions. Elderly people are being cared for by robot nurses, brewing a perfect beer using AI, interpreting medical test results, implementing industrial robots in workplaces, conducting audits and evaluating loans for customers in banks, digitizing textbooks, and kicking off students’ boredom using facial recognition, and AI customized travel planners are some of the upcoming developments in this adventurous AI realm. These advancements impact AI recruitment services as well, which is marked by advancements in NLP. Some of them are pre-trained language models, transfer learning, and multilingual models.
Conclusion
An elemental introduction to artificial intelligence and machine learning is sufficient to ignite an unquenched passion in the domain in willing minds and let them carve niches in the proliferating AI landscape. The ample scope for remarkable innovations in this sphere certainly impacts every field, including healthcare and finance. The inherent futuristic approach of AI and ML in every walk of life will bring forth indelible impressions now and forevermore.